Onfido, an Oxford University spin-out, launched the software amid surging identity fraud. Growing economic pressures, increasing digitization, and pandemic-fuelled upheaval recently led politicians to warn that a “fraud epidemic” is sweeping across Onfido’s home country of the UK.
Similar developments have been observed around the world. In the US, for instance, around 49 million consumers fell victim to identity fraud in 2020 — costing them a total of around $56 billion.
These trends have triggered a boom in the ID verification market. Increasingly sophisticated fraudsters are also forcing providers to develop more advanced detection methods.
Onfido gave TNW an exclusive demo of their new entry to the field: a head-turn capture experience dubbed Motion.
This was the sweet spot.
The uptake of biometric onboarding has been curbed by two key problems. “Active” detection methods, which ask users to perform a sequence of gestures in front of a camera, are notorious for high abandonment rates.
“Passive” approaches, meanwhile, remove this friction as they don’t require specific user actions, but this often creates uncertainty about the process. A little bit of friction can reassure customers — but too much scares them away.
Motion attempts to resolve both these issues. Giulia Di Nola, an Onfido product manager, told TNW the company tested more than 50 prototypes before deciding that head-turn capture provides the best balance.
“We experimented with device movements, different pattern movements, feedback from end users, and work with our research team,” she said. “This was the sweet spot that we found was easy to use, secure enough, and gives us all the signals we needed.”
Onfido says the system’s false rejection and acceptance rates are under 0.1%. The verification speed, meanwhile, is 10 seconds or under for 95% of users. That’s quick for onboarding clients, but fairly slow for frequent use — which may explain why Onfido isn’t yet using the service for regular authentication.
In our demo, the process felt swift and seamless. After sharing a photo ID, the user is directed to provide their facial biometrics via a smartphone. They’re first instructed to position their face within the frame and then to turn their head slightly towards the right and the left — the order doesn’t matter.
As they move, the system provides feedback to ensure correct alignment. Moments later, the app delivers its decision: clear.
Under the hood
While the user turns, AI compares the face on the camera with the one on the ID. The video is sequenced into multiple frames, which are then separated into different sub-components. Next, a suite of deep learning networks analyzes both the individual parts and the video in its entirety. The networks detect patterns within the image. In facial recognition, these patterns range from the shape of a nose to the colors of the eyes. In the case of anti-spoofing, the patterns could be reflections from a recorded video, bezels on a digital device, or the sharp edges of a mask. Each network builds a representation of the input image. All the information is then aggregated into a single score. “That is what our customers see: whether or not we think the person is genuine or a spoof,” said Romain Sabathe, Onfido’s applied science lead for machine learning. Onfido’s confidence in Motion derives, in part, from an unusual company division: a fraud creation unit. In a location that resembles a photo studio, the team tested various masks, lighting resolutions, videos, manipualted images, refresh rates, and angles. In total, they created more than 1000,000 different examples of fraud — which were used to train the algorithm. Each case was tested on the system. If it passed the checks, the team probed Motion further with similar types of fraud, such as different versions of a mask. This generated a feedback loop of finding issues, resolving them, and improving the mechanism. Motion also had to work on a diverse range of users. Despite stereotypes about the victims, fraud affects most demographics fairly evenly. To ensure the system serves them, Onfido deployed diverse training datasets and extensive testing. The company says this reduced algorithmic biases and false rejections across all geographical regions.
Plotting fraud
Sabathe demonstrated how Motion works when a fraudster uses a mask. When the system captures his face, it extracts information from the image. It then represents the findings as coordinates on a 3D chart. The chart is comprised of colored clusters, which correspond to features from both genuine users and types of fraud. When Sabathe puts the mask on, the system plots the image on the fraud cluster. As soon as he takes it off, the point enters the genuine cluster. “We can start understanding how the network interprets the different spoof types and the different genuine users that it sees based on that representation,” he said. Onfido’s head-turn technique resembles one revealed last month by Metaphysic.ai, a startup behind the viral Tom Cruise deepfakes. Researchers at the company discovered that a sideways glance could expose deepfake video callers. Di Nola notes that such synthetic media attacks remain rare — for now. “It’s definitely not the most common type of attack that we see in production,” she said. “But it is an area that we are aware of and that we are investing in.” In the field of identity fraud, both attacks and defenses will continue to rapidly evolve.
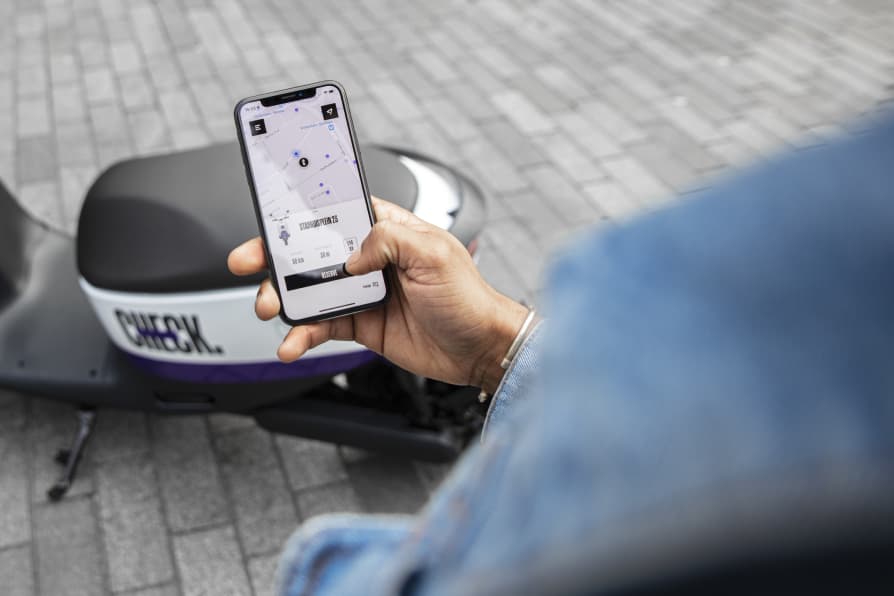