Their tests suggest the models can accurately predict attacks in regions that are already affected by terrorism. However, they found that “black swan events,” which occur sporadically, are almost impossible to predict. The researchers, led by Dr Andre Python from Zhejiang University in China, used publicly available data to analyze the location and data of attacks that occurred between 2002 and 2016 in 13 regions of the world. “We define terrorism as politically motivated attacks outside legitimate warfare (i.e., targeting noncombatants) perpetrated by non-state actors to communicate to a wider audience,” the group wrote in their study paper. Predictive models were built for each of the individual regions. The algorithms incorporated numerous variables that could make them targets for attacks, from proximity to international borders to population density. The team said their models can produce interpretable and accurate predictions of attacks a week ahead in areas with fairly high levels of terrorism. The results showed how the probability of an attack changes over time. In regions strongly affected by terrorism, such as Southeast Asia, the risk of a terrorist event peaked roughly 200 weeks after a previous attack. In areas with a lower terrorism prevalence, such as Russia and Eastern Europe, the risk continued to increase until around 400 weeks. Per the study paper: The team also found that the probability of terrorism tends to be negatively associated with population density: You can read the open-access study paper in the journal Science Advances. This story is adapted from the Neural newsletter. You can subscribe to it right here.
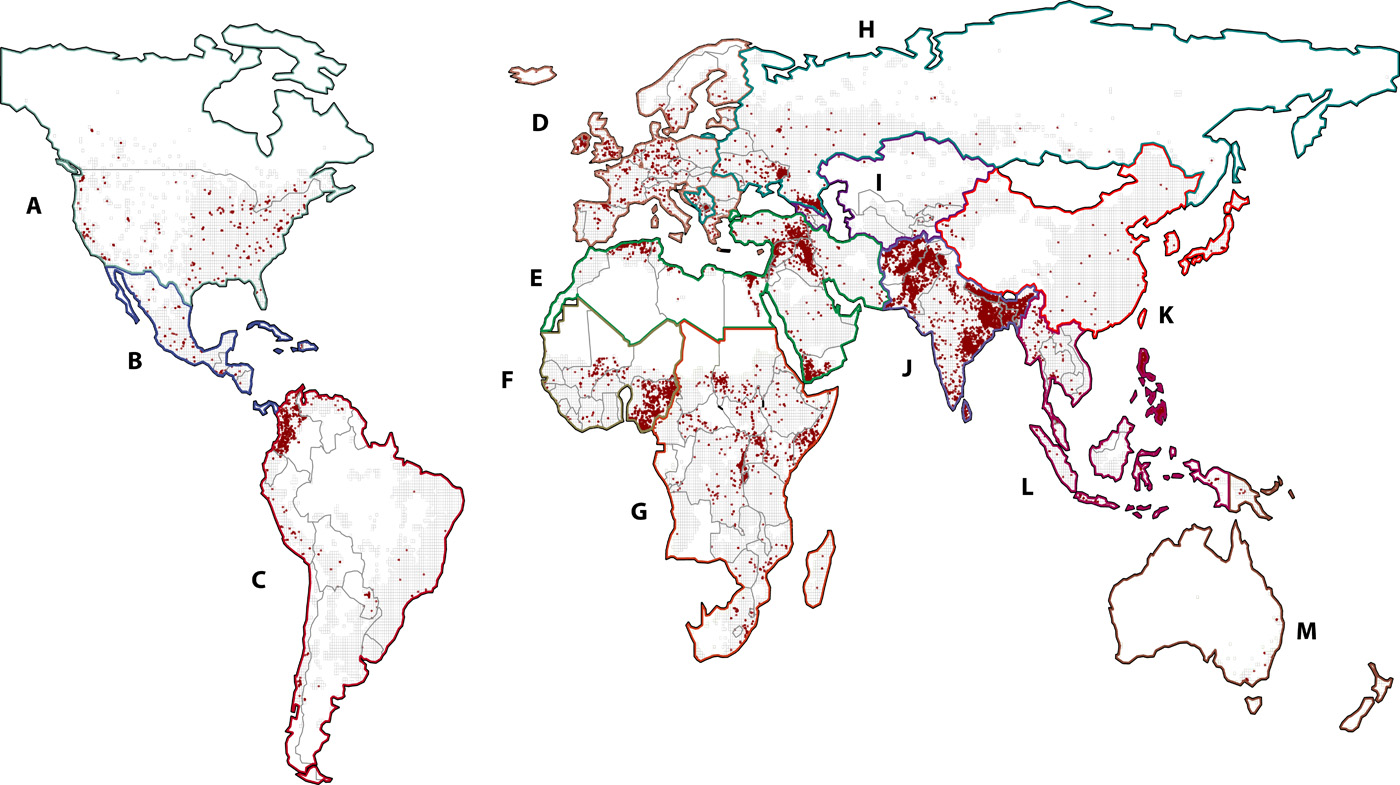